RESEARCH
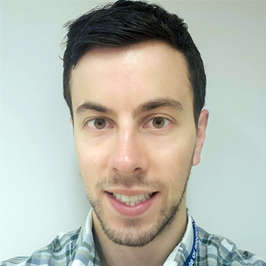
Artificial Intelligence to Generate Synthetic Pathology Images

Over the past decade, artificial intelligence techniques (AI) have been applied to a range of medical tasks, including radiographic image analysis, early detection of acute kidney injury, and the diagnosis of genetic syndromes from facial features. While prior AI methods had challenges in analyzing image and text data, recent advances in a form of AI called deep neural networks has revolutionized the field and led to expert level performance in a number of medical specialties. In pathology, AI has shown great potential in a range of tasks, including identifying cancer, predicting patient outcomes, and classifying genomic driver mutations and molecular subgroups solely from images. One area that has remained relatively unexplored is the synthesis of pathology images, motivated by numerous potential uses in medical education, clinical quality assurance, data sharing, and in augmenting data sets for training other AI models.
A team led by Drs. Adrian Levine, Blake Gilks, Stephen Yip, and Ali Bashashati used a type of AI model called generative adversarial networks (GANs), to synthesize high resolution, realistic histopathology images. GANs consist of a generator network (analogous to a counterfeiter), which creates synthetic images, and a discriminator network (the detector), which takes as input the synthetic images, as well as a set of real training images, and attempts to determine which are real and synthetic.
Levine et al. trained GANs using small image tiles extracted from whole slide images (WSIs) of pathology slides scanned at 40x objective. Models were trained to synthesize a wide range of distinctive morphologic characteristics (corresponding to 5 cancers: low-grade glioma, hepatocellular carcinoma, lung squamous cell carcinoma, renal clear cell carcinoma, and papillary thyroid carcinoma) as well as subtle morphologic differences corresponding to the five main histotypes of ovarian carcinoma (high-grade serous, low-grade serous, endometrioid, clear cell, and mucinous).
To evaluate the quality of the synthetic images the team constructed surveys (available at: http://gan.aimlab.ca/) to facilitate their evaluation by consultant pathologists. The surveys included an equal number of synthetic and real images of the five cancer histotypes and the five ovarian cancer histotypes. Interestingly, results demonstrated that for both data sets, the synthetic images are indistinguishable from real images, are rated as equally high quality, and are classifiable with equivalent accuracy. To further evaluate the quality of the synthetic images, as well as establish their value in building AI models for histotype classification where limited data is available, the team demonstrated that the addition of synthetic images improved AI classifier accuracy similarly to the improvement that one would see with the addition of real images.
Taken together, for the first time this study shows that GANs can synthesize a wide range of high resolution cancer pathology images that are indistinguishable from real images to expert observers and are useful as training data for other AI algorithms. The synthetic images have numerous applications, ranging from those that are directly and immediately clinically relevant (e.g., for quality assurance), to others that are more research-focused and technical in nature (e.g., data sharing between institutions and federated learning). The team is therefore optimistic that advances in generative modelling in medicine are an important step in the implementation of clinical artificial intelligence systems for improved efficiency and safety of care.
The authors have created a publicly available website where clinicians and researchers can attempt questions from the image survey at http://gan.aimlab.ca/. The manuscript has been published in the Journal of Pathology and can be accessed at https://doi.org/10.1002/path.5509.
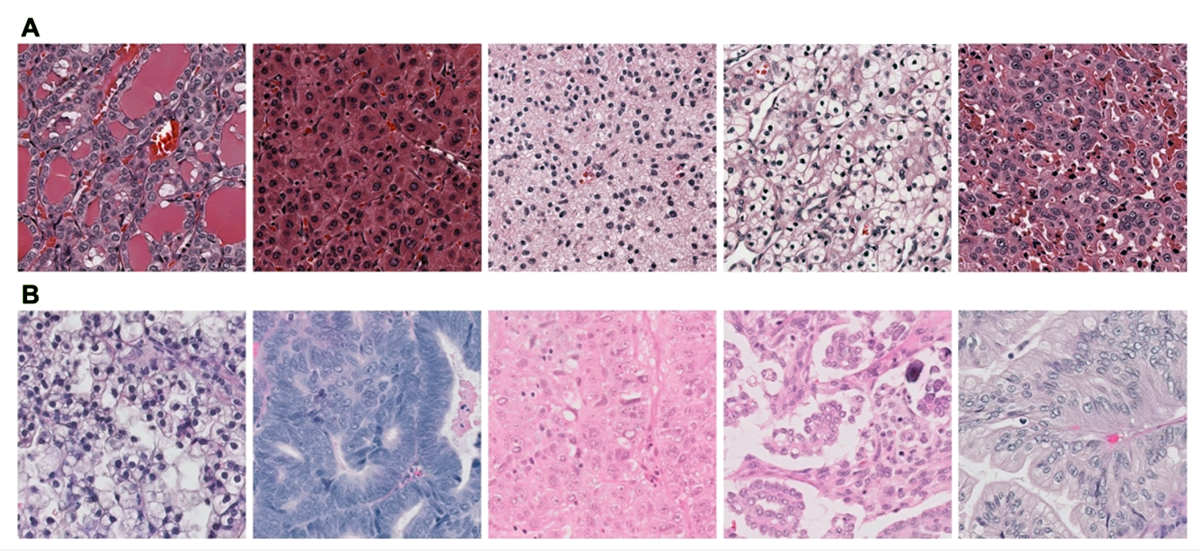
(from Levine AB, Peng J, Farnell D, et al. Synthesis of diagnostic quality cancer pathology images by generative adversarial networks. J Pathol [Internet] 2020;Available from: http://dx.doi.org/10.1002/path.5509)